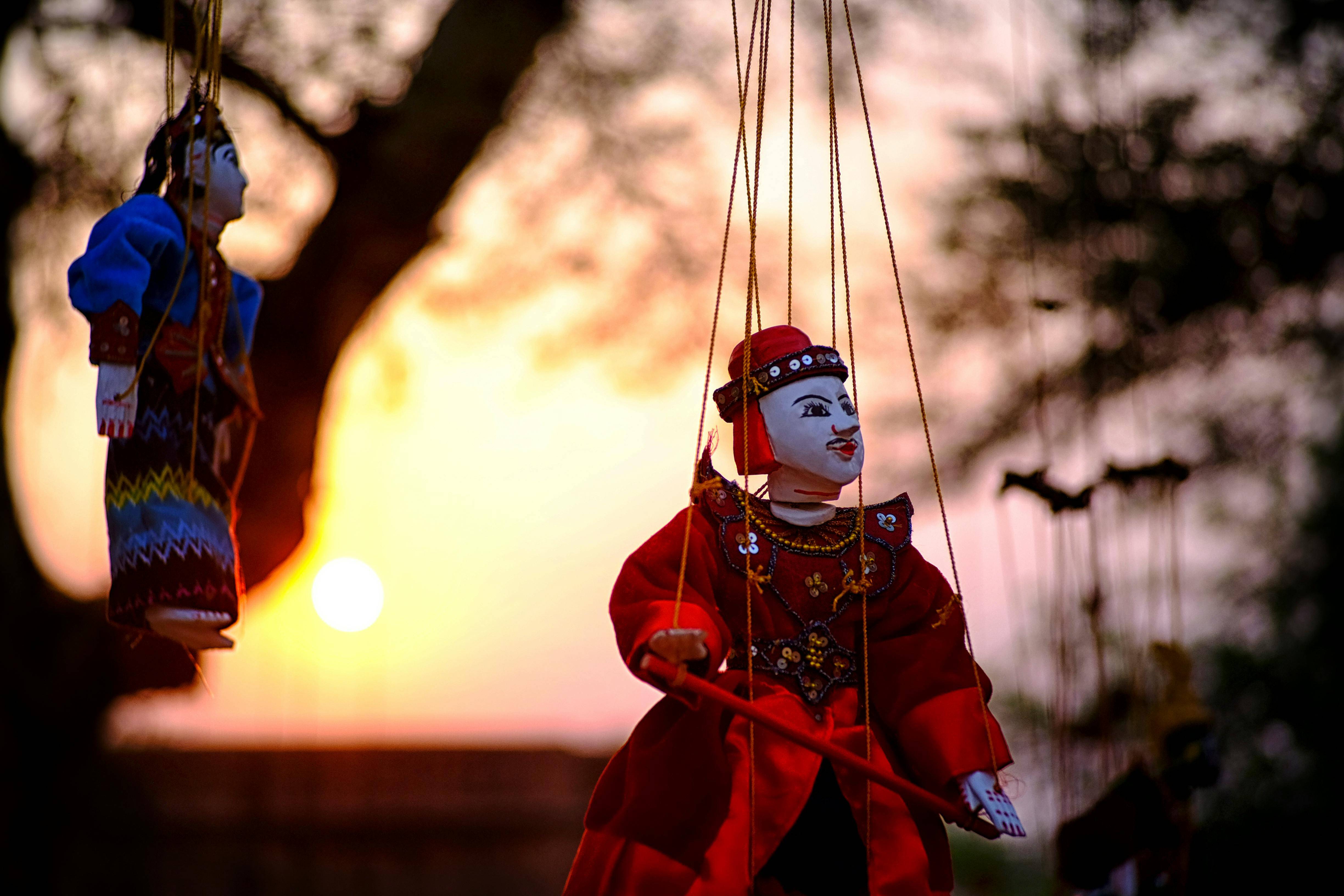
The Autonomy Paradox

We need a new framework for thinking about user choice, agency, information and how people can get the best and safest experience from online content.
The autonomy paradox is: you need information to make good choices; but first you have to choose what information to get.
The principle of autonomy is a powerful one and receives justified precedence in decisions about regulation, nudging, choice architecture and consumer welfare. According to the principle, people should have the freedom to choose inputs (such as products, information or services) that, in their own judgement, will help them achieve their goals.
But a consideration of the process they must go through to make such choices demonstrates a paradox that exists on several levels.
- "achieve their goals": what goals does a person have? Do they know what they are? Do they choose their own goals or are they influenced to prioritise certain things?
- "in their own judgement": is their judgement reliable? Can it be aided by external tools? Do people know their own biases?
- "choose inputs": what range of inputs is available and known to the chooser? Are all inputs equally available and neutrally framed?
- Meta-goals and meta-judgements: what if one of the person's goals is to reduce choice fatigue? Are they obliged to make more choices than they want to?
I propose a new theoretical framework to address this paradox. Apologies in advance for some technical language, but I am sure regular readers of this blog will understand.
Developing a model of consumer choice, welfare and harm
A model of consumer welfare requires some metric by which to estimate or compare welfare outcomes. In most economics this measure is called utility, and arises from satisfaction of a set of preferences over goods and services. Information economics extends the idea of preferences to preferences over information (e.g. Ho, Hagmann and Loewenstein 2021).
An alternative, neuroeconomics-based, approach uses mental reward, typically thought to be correlated with neurochemicals such as dopamine and serotonin, as the ‘currency’ for both consumer choice and consumer welfare. In these models there is no expectation of time consistency: a person’s choices at one time may result in higher reward at the moment of choice, but lower reward in the longer term.
Here we consider consumer choice in a social media environment using both models, as a lens to better understand how these environments can serve consumer interests.
Preference-based model
In a preference-based model, what might the important preferences be for a person consuming content online? Work by Loewenstein & Golman (2015) and Dan et al (2020) suggests consumers have a preference for curiosity satisfaction. Much other work confirms preferences for knowledge, for aesthetics and entertainment – the main elements of media “consumption”. These preferences are discounted over time at a consistent rate, and traded off against the costs of satisfying them, including the hourly value of the user’s time (the labour rate) and any financial costs.
Following this model, we could analyse user choices as follows:
- A user sees a post that provokes curiosity to click on a link or thread
- They do not yet have information about what will be revealed when clicking
- The content found in the thread has negative utility (or insufficient positive utility to justify the time cost of reading it)
- The user’s overall welfare is reduced, but the platform has gained readership and advertising exposure
Because the user has limited information prior to opening the link or thread, they are not able to accurately predict the utility of opening it. Only the utility of curiosity satisfaction can be predicted, and that ‘traps’ them into a negative experience.
Neuroeconomics/reward model
In this model, there is no time-discounted preference to consider at the time of choice. Instead, the brain uses simulation of outcomes to make affective predictions of the tree of causal consequences it can foresee from choosing each potential option.
The tree of consequences might look like this (sorry about the maths – it’s just there to show how we might calculate the outcome):
- The user sees a post that creates an information gap (for example, by promising “the five secrets you need to know about losing weight”). This creates a choice with uncertain outcomes: the option to ignore the link but be aware of the existence of new information about weight loss; and the option to click on the link and find out the five secrets (which themselves are unknown, with their reward value difficult to estimate). The resolution of that uncertainty is promised by clicking.
- Option A: click on the link
- Consequence A1 [probability p1]: Find out five useful secrets [positive reward r1]
- Consequence A1a: Use several minutes of time [negative reward r1a]
- Consequence A2 [probability p2]: Find out some useful information although not as much as promised [positive reward r2]
- Consequence A2a: Use several minutes of time [negative reward r1a]
- Consequence A3 [probability p3]: Be exposed to information you already know [zero reward]
- Consequence A3a: Use several minutes of time [negative reward r1a]
- Consequence A4 [probability p4]: Be exposed to information that is harmful or unpleasant to view [negative reward r4]
- Consequence A4a: Use several minutes of time [negative reward r1a]
- Consequence A4b: Negatively-rewarding replay of memories, e.g. a traumatic image coming to mind involuntarily [negative reward r4b]
- Option B: don’t click on the link
- Consequence B1: Be aware for the next few minutes that there is information available that you don’t have access to [negative reward rb1]
- Consequence A1 [probability p1]: Find out five useful secrets [positive reward r1]
The user’s brain evaluates this tree of options to estimate the highest-reward outcome in order to make its preferred choice. The “expectation value” of each option is the predicted reward, weighted by the probability that each branch will occur. If p1(r1+r1a) + p2(r2+r1a) + p3(r1a) + p4(r4+r1a+r4b) > rb1, the user will click; otherwise, they won’t. Very logical, except that some biases may enter into this evaluation:
- The content of the original post may bias the probability estimates (p1, p2, p3, p4) increasing the estimated value of p1 above its true objective value; especially true since the user does not spend long enough deliberating to develop accurate, objective probability estimates
- The estimate of reward r1 may be upwardly biased by its greater salience (evoked by the promise of the post)
- The estimate of negative reward r1a may be downwardly biased by being of unknown length, and by being causally downstream of the potential rewards r1 and r2
- The estimate of probability p4 or the estimated size of negative reward r4b may be upwardly biased by previous negative experiences, or downwardly biased by complacency about the safety of the environment. It is known that very low probabilities are typically underestimated by consumers, potentially because most of them have zero examples of these low-probability events in the sample they can retrieve from memory.
The first three biases, in this example, make the user more likely to click on the post, even if the expectation value of reward is lower for option A than option B. (The fourth bias could go either way.)
A more general model
A stream of posts {pn} is made available to a user U. Engaging with, reading and fully consuming pn produces reward rn (or, if we use the preference model, actual utility un). However, prior to engaging with the post, U makes a prediction of the anticipated reward from the post, a prediction that depends on the content of the post itself but also on U’s beliefs {belU}, the way the post is presented (pres) and the characteristics of the population of posts from which it is drawn: pred(pn, {belU}, pres, {pn}).
The platform’s quality of alignment can be defined as the statistical correlation between pred(pn, {belU}, pres, {pn}) and rn (for some suitable choice of correlation function).
Higher quality of alignment is a sign that users are getting what they expect, and are therefore able to exercise informed autonomy. This preserves user freedom without requiring a regulator to make controversial decisions about specific types of content. (Of course, the regulator may reserve the right to make additional rules for some types of content either because they can cause harm to third parties, or because informed autonomy is not a sufficient guardian against all forms of harm).
Design and regulatory response
In this example, the social media platform has an incentive to prioritise curiosity-provoking posts in its feeds. These will result in higher engagement and more clicks from users, as well as the likelihood that higher attention will be given to advertising alongside the content.
This example is not far-fetched; indeed the phenomenon of curiosity-provoking posts or headlines that do not fulfil their promise is common enough to have its own name, clickbait.
Users’ interests may well not be served by clickbait; just because people choose to click on a post does not mean that they get a positive experience from doing so.
Clickbait is a prime example of the autonomy paradox. Users have the free choice to click, or not click, on a link. Many of them choose to click; but are dissatisfied with the results. Indeed, the introduction of clickbait headlines reduces the probability that users will have a positive experience, while increasing the number of engagements. The choice to click does not necessarily serve users’ interests – not because of their freedom to choose, but because the context in which choices are offered to them, and their own attentional filters, prioritise and make salient options that are not aligned with their interests.
An alternative design that better supports user interests might incorporate:
- Prioritising posts whose threads have been positively rated or liked by users (suggesting that they received positive outcomes from clicking), and downweighting those that have been negatively rated
- An algorithm that gives lower priority to posts that people merely clicked on, without liking the subsequent posts in a thread
- An option for users to mark a post as ‘clickbait’ to help other users calibrate their expectations of what they’ll see when they follow the link
- Features that offer greater lookahead allowing users to see what they’ll get before clicking: for instance, a post feed that shows the first reply to a post in the main feed rather than requiring a click; or post statistics that reflect positive experience rather than simply showing ‘the most clicks’.
- Other measures to increase quality of alignment, as measured by the correlation proposed above.
The regulator’s role may be to evaluate users’ experiences and encourage the adoption of design features that give users a real autonomy. Not a conditional autonomy moderated by the choice architecture offered to them, but an empowered autonomy that allows meaningful choices over the experiences and kinds of knowledge that they would like to consume.
References
Dan, O., Hassin, R., & Leshkowitz, M. (2020). Preference reversal in curiosity-based information consumption.
Golman, R., & Loewenstein, G. (2015). Curiosity, information gaps, and the utility of knowledge. Information Gaps, and the Utility of Knowledge (April 16, 2015), 96-135.
Ho, E. H., Hagmann, D., & Loewenstein, G. (2021). Measuring information preferences. Management Science, 67(1), 126-145.