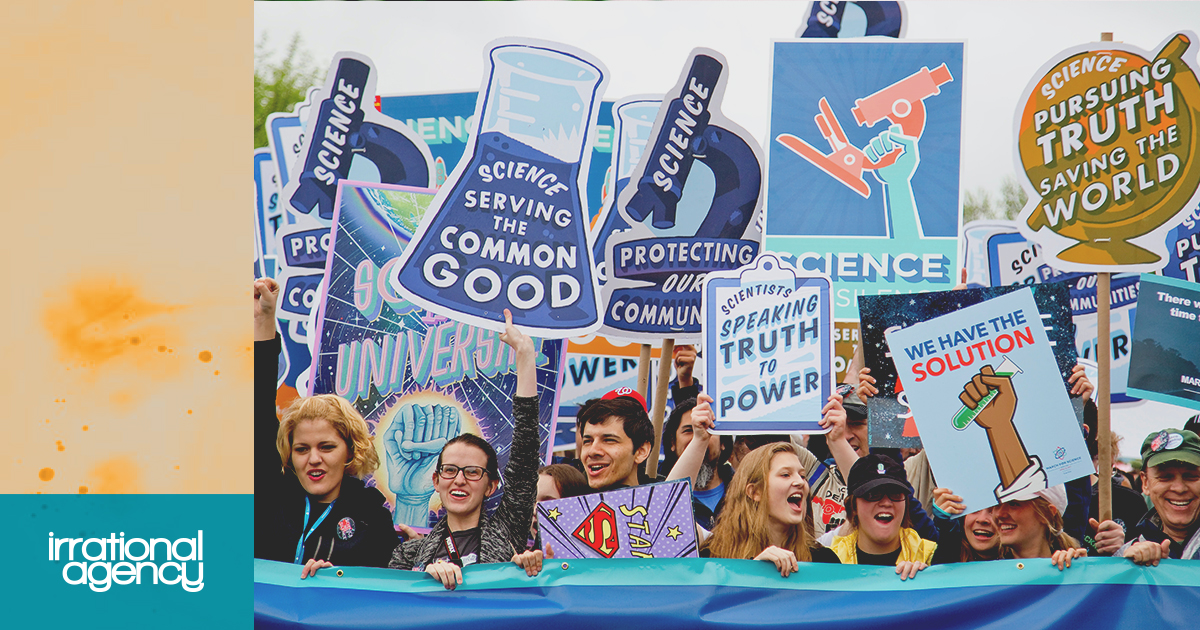
What is the Role of Behavioral Science in a Pandemic?

This moment is a high-profile test for behavioral science. The UK government has drawn a clear line between its policy and those of governments in Asia and elsewhere in Europe, deciding not to immediately close down schools and ban large events. Its primary rationale is advice from behavioral scientists that acting too early could result in 'fatigue.' If we all have to stay at home now, maybe we won't be willing to do so later when it may be more critical.
Although I am more confident than some in behavioral science as a field, I wondered how the government is using it during this crisis. It turns out there are three different questions, and the answers are nuanced:
-
Can behavioral science improve individual actions that will save lives?
-
How do individual behaviors interact to lead to larger-scale social outcomes, and do behavioral scientists have something to contribute there?
-
Is the claim about the risk of public fatigue actually correct?
Hiding beneath all of these is a controversy about the nudge discipline as a whole: is it real science, does it actually work, and should it be used in situations like this? I'll come back to that.
The first question is the easiest.
The most obvious example in the current context is handwashing. If I wash my hands more, I can be confident that I'm less likely to catch the virus – or if I have it already, I'm less likely to spread it to someone else. This is called a monotonic relation: more handwashing unambiguously leads to less disease. Behavioral science is good at changing individual behaviors – think about those urinals. So yes, nudging might have a beneficial role in instilling better individual habits to improve public health.
One thing we can't know is how much difference more handwashing will make. There are probably no reliable statistics on the number of times people touch their face, the number of virus particles on each person's hand, the relationship between number of particles and chance of infection – this information can only be gathered by precise measurement and will vary for each new flu strain. Nor do we know how successful the interventions will be at increasing handwashing. So it will be hard to predict the impact of handwashing interventions – but it will be positive, and the downside is low.
In this case, behavioral science can definitively improve outcomes.
The second question – about the interactions of multiple people – brings in a different discipline: population modeling, a subfield of complex systems study. Here is where the mathematics of epidemiology comes in: transmission rates, incubation periods, flattening the peak, and all that. We go beyond individual behavior and start to ask how interactions between people lead to particular outcomes.
The handwashing example, along with many famous nudges on issues like paying tax on time or organ donation, is an area where interactions between people are not very important. My propensity to wash my hands doesn't much affect yours (maybe just a little, if we're in the bathroom at the same time.) It is primarily a one-to-many interaction: the government (one) does something, and people (many) respond. The mathematics of one-to-many situations is quite simple. If 80% of people usually pay their tax and you can increase it to 90%, tax revenue will go up by ten percentage points.
When many-to-many interactions come into play, you can no longer use simple statistics to scale up from a test and forecast the outcome. If 80% of people were previously vaccinated against measles, and you can push it up to 90%, the spread of the disease may be stopped altogether – or it may make no difference at all. The outcome depends not just on the change in total number of vaccinated individuals, but on the connections between people, how often they meet, the likelihood of spreading from one to another, the incubation period…all of these variables have to go into the model and their interactions are complex and non-obvious – hence the name, complex systems.
You need population models to predict these outcomes – the specialism of epidemiologists and public health professionals. The massive challenge of this approach is that what goes into the models determines what comes out.
How often do individuals meet each other in daily life? How much close contact is there when people attend a conference together? A football match? If a sick person is at the conference, what is the probability they will infect others – and how many? All of those data points have to be entered into the model and will make a big difference to what the model predicts, as well as a big difference in the real world. The chasm between death rates in Italy and deaths so far in France, Spain or Germany could be explained by a hidden micro-level variable like this and we might never know.
(The question of 'herd immunity' is also in this domain. It is hard to predict what prevalence of flu in the population will slow the transmission of the disease: because the prevalence itself, and people's perception of it, will change their behaviors. 50% immunity might be enough this summer, but it could require 80% once behavior reverts to normal.)
Some of these variables can be calculated from past experience. Others are unmeasurable but can be estimated. This is where behavioral science comes back into the picture. If you have no data on a question, such as how many interactions someone has at a conference, there are two approaches. You could guess – or you could use insight and judgment from people who study human behavior to come up with an informed estimate.
Here, behavioral scientists have to be appropriately humble. Expertise is valuable, but it doesn't guarantee correct answers. Indeed, this is why the behavioral science discipline is so keen on running tests and experiments – we know that human interactions are hard to predict, so a hypothesis could easily be wrong or be drowned out by other factors.
However, when there is no time to run an experiment, it's better to have some insight and experience than none. In practice, the alternative to having a behavioral scientist give an estimate of one of these variables is to have a programmer make up a plausible-sounding number to plug into the model. I'd prefer to have the expertise.
The answer to the second question, then, is: use data to populate these models where we have it; but where we don't, behavioral insights have a role to play.
The third question – is the 'fatigue' advice correct? – leads us into the most complex and controversial area: the idea of non-monotonic functions. Monotonic functions like handwashing are relatively easy to introduce into your model: when more people wash their hands, there is less spread of the disease. The policy advice is unambiguous: get people to wash their hands.
Not all variables are like that. For example, should people go to the hospital if they have flu symptoms? If there are only a few cases, the answer is yes: it's better to identify infected people and, if necessary, isolate them in a safe environment. If there are many cases, the answer is no: too many people in the hospital will overwhelm the available beds and spread the disease to more vulnerable people. Somewhere in the middle is a transition point: below that point, we should advise people to go to the hospital; above it, advise them to stay at home.
These questions are where the population models are most sensitive – and most at risk of going wrong. To choose the right policy, it's critical to know whether we are above or below that transition point. The right expertise could make a big difference in estimating that point.
The people best placed to answer the example above – about hospital spreading of infections – are epidemiologists and people who run hospitals. They understand how individuals interact within those spaces, they have historical data on the spread of infections and viruses in hospitals, and that information is very useful in calibrating the models.
But epidemiologists don't have such detailed historical data on interactions outside of hospitals. In those areas, other expertise might be needed.
"Another risk is imposing restrictions too early in the outbreak, leading to people becoming fatigued and ignoring instructions when it matters." David Halpern from here
This leads us to the most controversial UK policy at present – the idea that we should wait longer before introducing social distancing because people will get fed up with it and stop obeying the guidelines. The claim is that this is another non-monotonic function – where more distancing and more draconian measures do not necessarily lead to better results.
The population modelers have probably built a model containing a 'compliance' variable. If they can predict how compliant people will be and how that will change over time, they can make forecasts of the spread of the disease and choose the best policy. If that compliance variable is genuinely non-monotonic – for example, if greater compliance now implies less compliance later – then it's imperative to understand that.
So this is another case where – in the absence of data from past epidemics – we need another way to estimate the compliance variable. The fatigue claim has been described as a recommendation from behavioral science. Is it? And does it have evidence behind it?
It's not clear that anyone has good data. It's hard enough to collect that kind of data in a stable, replicable environment: this 2009 paper by Murphy et al discusses predictors of compliance (women and ill people are more likely to comply than men and healthy people), but doesn't touch on whether compliance changes over time. (The authors of that paper are a mix of public health specialists and researchers in communication strategies – a behavioral discipline.)
A 2009 study in Australia by Hunter et al explored changing compliance over time and found that compliance would increase as an emergency becomes more serious, due to a greater perception of risk. This study specifically asked if people were willing to comply with social distancing measures for a period of one month. The study was a telephone survey based on what people said they would do in a pandemic, not on observation of real behaviors.
There is some information from a key historical source – the 1918 flu epidemic that killed 40 million people. Markel et al (2007) analyzed the details (via Marginal Revolution). In some cities such as Denver, there was a double peak of social distancing measures – a first round of school closures and other measures was relaxed and then enforced again later, resulting in a double peak of the disease. However, this paper still does not tell us whether people become less willing to comply with instructions over time.
Rothstein & Talbott (2007) look at the question from an economic point of view: do people need to be compensated for lost income in order to remain in quarantine? The simple answer: yes. This (2020!) CDC study by Fong et al looks (among other things) at the effectiveness of larger-scale social distancing measures such as banning large gatherings, but mainly a literature review which in turn relies on the same Markel et al paper mentioned above.
Another academic study, Collinson et al (2015) is probably the closest to answering the question. It finds that: "media fatigue can also produce two waves of infection with similar qualitative dynamics to that observed over the 2009 H1N1 pandemic". However, this is about fatigue from media coverage, not government instructions. The effect on social distancing is still uncertain: "A study of the effects of the type of mass media message on social distancing behavior is greatly needed, and is a course for future work."
Finally, Karla Vermeulen, a psychologist in New York, wrote a 2014 article about how public emergency officials can improve compliance by getting their messaging right. This is an article based on applying psychological theory, discovered in other contexts, to a new field. The author admits that "…specificity inevitably results in complexity that makes each theory virtually impossible to actually apply in crafting a specific warning" – not a very hopeful prognosis. But she does suggest four principles that could help.
So to make any predictions at all, the modelers will likely – once again – have to make some assumptions about compliance changes over time. Those assumptions could be based on guesswork, or they could be based on the considered judgment of people who understand the psychology of compliance. Behavioral scientists might well, once again, have a role to play in this.
It's plausible that compliance might rise and fall – but it's not possible to say definitively that it will. It's also plausible that the opposite is true: once people find successful workarounds (remote working tools, home shopping delivery), they could get better at social distancing over time. The argument should not be based on plausibility but on proper analysis.
If the government and the Behavioral Insights Team have evidence for the fatigue claim, or have identified published papers that support it, they should share them. Transparency, debate and challenge are the best ways to make these findings as robust as they can be.
We return to the controversy about behavioral science. Transparency, debate and challenge is exactly what didn't happen enough in the 'replication crisis' – the discovery in recent years that some findings of social psychology did not hold up when re-tested. A few of the problems originated in deliberate faking of data, more of them in careless or biased data analysis, but the most important lesson from that era was that behavioral effects often work in one context but not in another. This makes it hard once again to extrapolate from historical data to the current situation – but data from another time and place is still better than no data at all.
For me, the replication crisis does not diminish the value of behavioral science. One reason is that it did not really apply to public policy nudges such as those run by the nudge unit, but was mainly about social psychology experiments such as "priming", run in university labs. Another is that the field has taken the issues very seriously and now requires a new level of statistical rigor, including pre-registration of experiments, public sharing of analysis programs, new ethical standards, and other measures.
And it's ethics that can answer the deepest question here: is it right to use behavioral science in this way? It is not a purely technocratic discipline because it deals with human wants and outcomes. Science is not only a matter of finding out what works better: because there can be no objective definition of "better." The fact unacknowledged in yesterday's press conference is that some very real tradeoffs are being made.
The truth is that this is not a purely technical question. It is not just about determining whether social distancing measures are monotonic. The government is not delaying these measures solely because of the risk of fatigue. It is delaying them because they would be hugely disruptive to the economy and to everyone's daily lives.
It is absolutely legitimate to take economic factors into account – even if your only priority were public health, recessions themselves cause more deaths and worse health outcomes and should be avoided. But the tradeoffs need to be acknowledged.
Tradeoffs are, in fact, another source of non-monotonic behavior: the tradeoffs and resource constraints in a model are some of the things that lead to unpredictable results. If there were no tradeoffs, the government could shut everything down now and keep them locked down for two months until the transmission of the disease is definitively over. It seems implausible that fatigue would prevent this from working.
This would probably save lives at the cost of major economic depression – but a decision has clearly been made that it would not be worth it. That's okay – governments have to make life-or-death tradeoffs all the time. That is the nature of politics. But to hide behind science and pretend they're not consciously making any tradeoffs at all is wrong and will end badly.
It is necessary that we question the government about its motives, about its economic advice, and about its medical advice. For example, the government might have a political incentive to gamble with the economy in hope that its 2020 GDP figures will outperform EU members who take more drastic action. They may intend to do nothing of the kind – but a behavioral scientist who has lived through the replication crisis would be aware of the dangers of confirmation bias and selective experimentation.
Behavioral science is a powerful tool and serves human goals and values. That means it is and should be subservient to politics, and democratically conducted. More disclosure of the government's values and goals is necessary to maintain the legitimacy of this process. Otherwise – if I may put forward a behaviorally informed but untested hypothesis – public fatigue may set in as we lose trust in the government's motives and advice.